Aditi Chandra1,2 and Marcelo O. Magnasco1
Phys. Rev. Research 6, 043152 – Published 18 November, 2024
DOI: https://doi.org/10.1103/PhysRevResearch.6.043152
The previous Arxiv submission has been published in Physics. Rev. Res.
We examine the dynamical properties of a single-layer convolutional recurrent network with a smooth sigmoidal activation function, for small values of the inputs and when the convolution kernel is unitary, so all eigenvalues lie exactly at the unit circle. Such networks have a variety of hallmark properties: the outputs depend on the inputs via compressive nonlinearities such as cubic roots and both the timescales of relaxation and the length scales of signal propagation depend sensitively on the inputs as power laws, both diverging as the input →0. The basic dynamical mechanism is that inputs to the network generate ongoing activity, which in turn controls how additional inputs or signals propagate spatially or attenuate in time. We present analytical solutions for the steady states when the network is forced with a single oscillation and when a background value creates a steady state of “ongoing activity” and derive the relationships shaping the value of the temporal decay and spatial propagation length as a function of this background value.
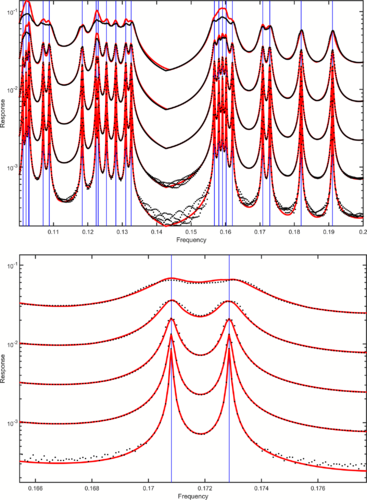
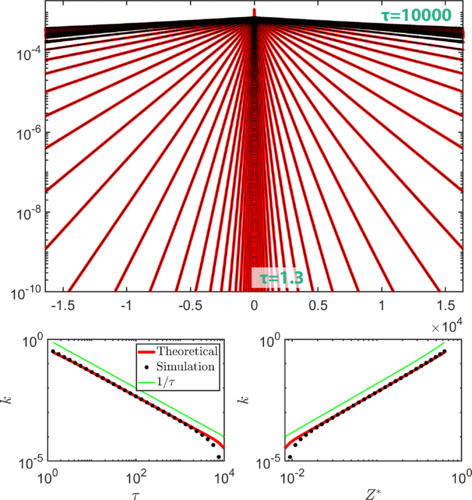